Karaoke heroics and cracking a good joke are both great reasons to use your voice, but the human voice is more than just a vehicle for communication — it’s a source of information about your physical and mental health. Every aspect of speech, from tone and pitch to rhythm and articulation, holds subtle clues about your neurological, respiratory, and emotional state.
Recent advancements in artificial intelligence (AI) and machine learning (ML) have unlocked the potential of voice biomarkers as a game-changing tool in healthcare. These biomarkers offer a non-invasive, cost-effective, and scalable way to detect and monitor various diseases. By analyzing voice patterns, clinicians can identify vocal biomarkers and spot early warning signs of Parkinson’s disease, cardiovascular disorders, and certain mental health conditions.
Voice-based diagnostics can change how healthcare professionals approach disease detection and patient monitoring. While traditional diagnostic methods often rely on invasive procedures or expensive imaging technologies, voice biomarkers offer an accessible alternative that can be integrated into telemedicine and mobile health applications. But how do voice biomarkers work?
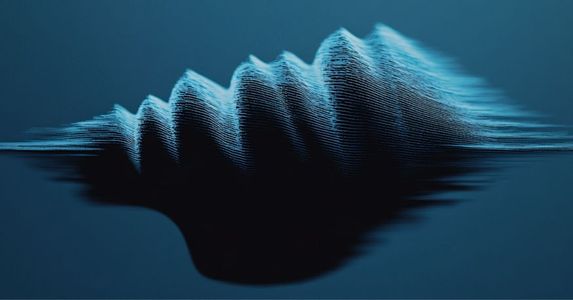
What are Voice Biomarkers?
A voice biomarker is a measurable characteristic in an individual’s speech that indicates the presence of a disease or medical condition. These biomarkers are derived from changes in pitch, tone, rhythm, articulation, and other acoustic features of the voice. Since voice production involves complex coordination between the brain, lungs, vocal cords, and muscles, subtle speech pattern deviations can indicate underlying health issues.
The Physiology of Voice Production
The human voice is produced by a combination of respiratory function, vocal cord vibration, and tongue, lips, and jaw articulation. The vocal cords, or vocal folds, are crucial in this process as they vibrate to produce sound waves. The process starts with air being pushed from the lungs through the larynx, where it causes the vocal cords to vibrate and produce sound waves. The vocal tract then shapes these sound waves into speech. Any disruption in this system — due to neurological conditions, muscular disorders, or respiratory diseases — can alter speech characteristics and can be detected through voice analysis.
How Voice Biomarkers Are Analyzed
Speech analysis uses advanced signal processing techniques combined with AI-driven algorithms to extract and interpret voice biomarkers. The process involves the following steps:
- Voice Recording: Patients record their voice by reading a passage, sustaining vowel sounds, or speaking spontaneously.
- Feature Extraction: Pitch, intensity, jitter (pitch variations), shimmer (amplitude variations), speech rate and pauses are analyzed.
- Pattern Recognition: AI and ML models compare the extracted features against a voice database to differentiate between healthy and affected individuals.
- Diagnosis and Monitoring: Based on statistical and machine learning models, deviations in voice features can indicate the presence of a specific condition to aid in diagnosis or disease tracking.
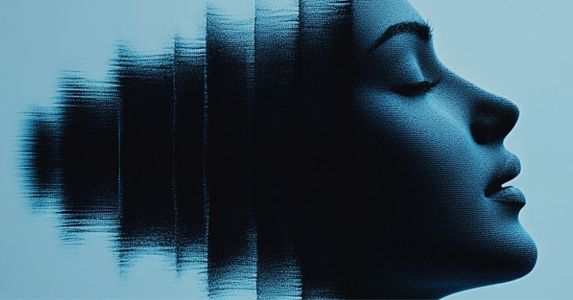
Identifying Vocal Biomarkers
Identifying vocal biomarkers is a crucial step in leveraging voice data for healthcare advancements. By pinpointing specific vocal features that correlate with various health conditions, researchers can develop tools for early diagnosis and continuous monitoring.
Data Collection and Machine Learning Techniques
The process of identifying vocal biomarkers begins with extensive data collection. This involves gathering voice recordings from individuals, particularly those with known conditions like Parkinson’s disease. These recordings can be obtained through various methods, including studio-based setups, telephone calls, web platforms, and smartphone apps. Each method offers unique advantages in terms of accessibility and convenience.
Once the voice data is collected, machine learning algorithms come into play. These sophisticated algorithms analyze the voice recordings to extract relevant features such as pitch, intensity, jitter, and shimmer. By comparing these features across a large dataset, machine learning models can identify patterns and anomalies that serve as potential vocal biomarkers. This process not only enhances the accuracy of diagnosis but also enables the development of automated voice biomarkers for continuous health monitoring.
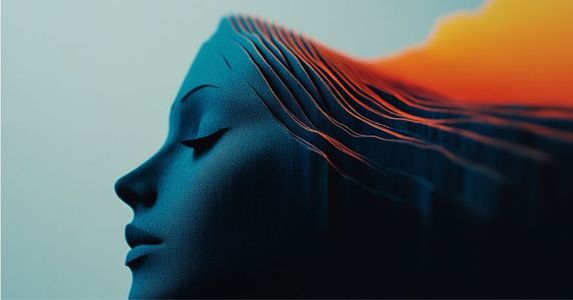
How Voice Biomarkers Can Be Used in Diagnostics
Neurological Disorders: Conditions like Parkinson’s disease, Alzheimer’s disease, and multiple sclerosis can affect speech due to their impact on motor control and cognitive functions. Voice biomarkers can detect early signs of these diseases, even before symptoms appear.
Mental Health Assessment: Depression and anxiety can alter voice patterns, tone, pitch, and speech rate. Voice analysis can provide objective markers for diagnosis and monitoring of these mental health conditions. People with depression often have reduced speech variability, lower pitch and more pauses. Anxiety can result in higher pitch and increased speech rate due to physiological arousal. By continuously analyzing these voice markers, AI-powered tools can track mental health trends over time and enable early intervention and personalized treatment approaches.
Respiratory and Cardiovascular Diseases: Conditions like COVID-19, chronic obstructive pulmonary disease (COPD), and heart irregularities can affect breathing patterns and vocal cord function, leading to detectable changes in voice biomarkers.
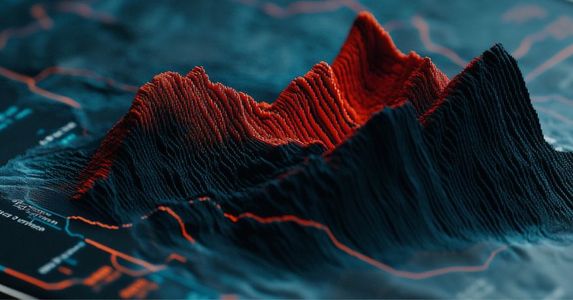
Biomarkers and Real-World Applications
The potential of vocal biomarkers extends beyond research and into practical, real-world applications. These biomarkers can revolutionize patient monitoring and digital health, offering new ways to manage and track health conditions.
Patient Monitoring and Digital Health
Vocal biomarkers are poised to transform patient monitoring, particularly for chronic conditions like Parkinson’s disease. By integrating voice analysis into remote patient monitoring systems, healthcare professionals can detect subtle changes in a patient’s condition without the need for frequent in-person visits. This continuous monitoring can lead to earlier interventions and more personalized treatment plans.
Digital health companions, such as virtual assistants, can also benefit from vocal biomarker technology. These companions can provide personalized health advice and monitoring based on the user’s vocal characteristics. For example, a virtual assistant could detect signs of stress or fatigue in a user’s voice and offer appropriate recommendations.
In clinical trials, vocal biomarkers offer a non-invasive way to monitor patient outcomes. By analyzing voice recordings, researchers can track the progression of a disease and the effectiveness of treatments. This approach not only enhances the accuracy of clinical trials but also reduces the burden on participants.
In conclusion, the integration of vocal biomarkers into healthcare systems holds immense potential. From early diagnosis to continuous monitoring, these biomarkers can lead to better patient outcomes and a more efficient system. As data collection and machine learning techniques continue to evolve, the future of vocal biomarkers looks promising.
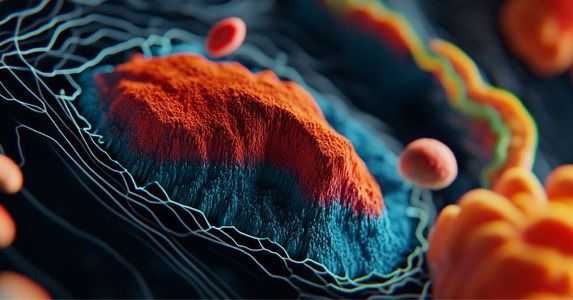
Decentralized Science Can Drive Voice Biomarker Research
While the potential of studying voice biomarkers is significant, there are challenges to overcome before clinical adoption. These include data privacy and security, improving AI models, and validating biomarkers across diverse populations. Regulatory approvals and integration with existing healthcare systems are also key. For this reason, the decentralized science ecosystem within a Web3 framework can play a crucial role in exploring voice biomarker assessments. Voice biomarker technology can be integrated into smartphones and wearables for continuous monitoring and personalized healthcare solutions.
For example, AxonDAO’s project A+ Voice is a great example of Web3’s role in biomarker assessments. Participants simply read a passage, and algorithms detect unique voice biomarkers. The data is then stored on AxonDAO’s native infrastructure, CureOS, so participants can continuously monitor their biodata. Participants even earn $AXGT, AxonDAO’s governance token, by simply lending their voice to science.
In the next few years, as AI evolves and voice data collection gets more sophisticated, voice biomarkers can play a large part in digital health. With the potential to revolutionize diagnostics, voice biomarkers can lead to earlier disease detection, better patient outcomes, and a more efficient healthcare system. Like DeSci in general, voice biomarker analysis is found at the intersection of medicine, tech, and AI, making this method of scientific research ideally suited for the decentralized science ecosystem.