Decentralized science (DeSci) has been a buzzword for a few years now — and for good reason. While some aspects of the movement remain more aspirational than applicable, the underlying intention of DeSci — to enable collaboration, transparency, and innovation in the scientific community — is steadily gaining traction. Overall, it’s up to innovators and problem-solvers to crystallize their visions into real-world DeSci use cases, and while magical thinking is easy, dream-into-reality alchemy is not. Fortunately, DeSci now wields a particularly powerful tool: artificial intelligence.
AI-driven analysis is one of the most promising applications in decentralized science. With the increasing complexity and volume of data generated in scientific research, DeSci requires advanced tools to process, analyze, and derive meaningful insights. For those without the luxury of institutional resources, AI has the potential to gap-fill this resource void – whether it’s related to personnel, time, or financing. In other words, for researchers operating within the DeSci space, AI could be the key ingredient for reality-making alchemy.
AI, especially machine learning (ML) and deep learning (DL) models, offers powerful capabilities to handle the data deluge that modern research creates. Also, considering the very nature of decentralized science, where scientific data itself is often spread across multiple nodes and researchers, AI becomes an essential tool to make sense of large, distributed datasets to help extract actionable insights.
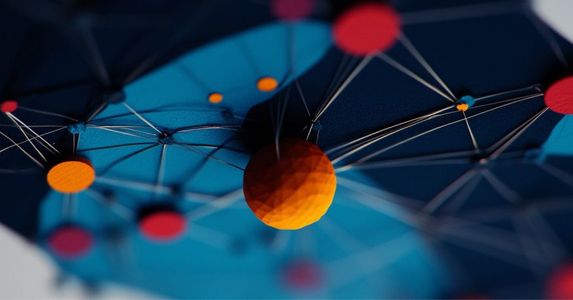
Decentralized Science: AI-Driven Data Analysis and Insights
In a perfect decentralized science world, vast amounts of scientific data will be generated and shared across open, distributed networks. Interpreting data has always been difficult, but the sheer amount of information in modern science is overwhelming. Fortunately, AI can be used to automate data analysis and help researchers find patterns and correlations in research data that would have gone unnoticed.
For example, AI models can be trained to detect anomalies, classify data, and perform predictive modeling. This is super useful in fields like genomics, climate science, and drug discovery, where researchers deal with massive datasets. And that’s just data within their own fields. Consider the researcher performing an interdisciplinary collaboration. They’re now trying to interpret huge and foreign-looking datasets in a meaningful way. By automating the data analysis process, AI helps reduce the time it takes to gain valuable insights.
AI can also help with meta-analysis by aggregating results from multiple decentralized research projects and identifying broader trends and conclusions. This ability to combine insights from different datasets improves research, allowing scientists to collaborate on large-scale projects and studies.
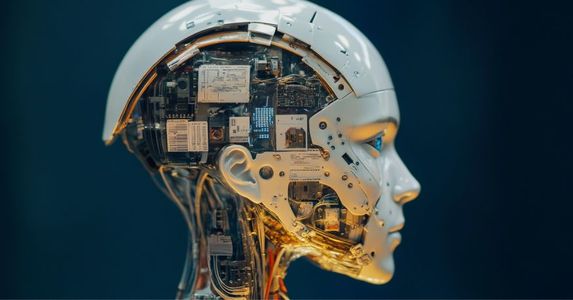
Collaboration, Data Sharing, and Global Connectivity in Scientific Research
One of the main goals of decentralized science is to create a more collaborative environment where researchers from all over the world can freely share their research data and resources. AI-powered tools can help with that. DeSci projects can benefit from this global connectivity, enabling researchers worldwide to collaborate on scientific knowledge on a scale never seen before.
For example, AI can facilitate communication across different disciplines by translating scientific jargon into layman’s terms or specialized terminology into other languages. This reduces the barrier to entry into scientific literature for researchers from different backgrounds and makes scientific knowledge more accessible.
AI-powered platforms can also recommend collaborators based on shared research interests and create networks of researchers with complementary skills and expertise. By unlocking scientific innovation, fostering collaboration, and using AI to connect researchers working on the same topic, DeSci platforms can help interdisciplinary research and accelerate scientific breakthroughs. Also, AI can monitor projects across decentralized networks and identify gaps or areas for improvement.
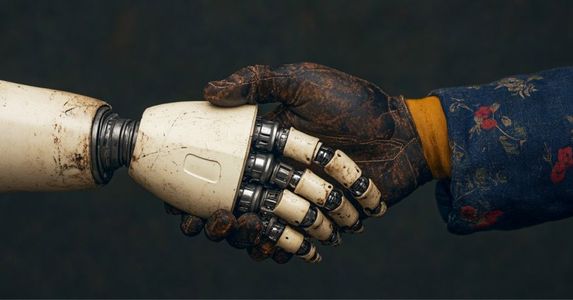
Automating Data Curation and Preprocessing
In decentralized science, data curation — the process of collecting, cleaning, and organizing data — is a time-consuming but crucial step. Many datasets in scientific research are incomplete, inconsistent, or noisy, requiring extensive preprocessing before they can be analyzed. Traditional science data curation often involves manual labor, which can slow down the research process.
AI can significantly reduce the time spent on data curation by automating many of these tasks. For example, machine learning models can automatically detect missing values, correct inconsistencies, or even normalize data for better compatibility between different datasets. AI can also be used to automate the classification of data, identifying the types of experiments or research methods that generated the data, which can make the data more accessible to researchers in other fields.
In decentralized science, where datasets are spread across different platforms and repositories, AI can automate the process of linking related datasets, identifying overlaps, and suggesting how disparate data can be combined to enhance the research process. By streamlining the data curation process, AI helps scientists focus on deriving insights rather than spending time cleaning and organizing their datasets.
Pattern Recognition and Anomaly Detection
One of AI’s key strengths, particularly for machine learning, is its ability to recognize complex patterns within data. In decentralized science, this capability can be used to identify meaningful correlations between different variables across distributed research projects. For instance, AI can help scientists detect emerging trends or relationships that might have been overlooked in smaller, isolated datasets.
Anomaly detection is another powerful application of AI in decentralized science. In research, an anomaly could represent an error, a novel discovery, or a potential breakthrough. AI algorithms can be trained to identify unusual patterns in data, whether it’s detecting fraudulent or erroneous data entries in research papers, identifying unexpected results in an experiment, or spotting new phenomena in observations. By flagging these anomalies, AI can help researchers avoid false positives or identify areas that warrant further investigation.
In fields like epidemiology, AI-driven analysis can also help detect outbreaks or unusual patterns in disease spread by continuously analyzing health data across multiple decentralized sources. This real-time ability to recognize patterns can save lives by facilitating quicker responses to emerging public health crises.
Predictive Analytics and Modeling
Predictive analytics is another powerful tool that AI brings to DeSci. AI models can be used to predict future events, trends, or outcomes based on existing data. For example, in drug discovery, AI-driven predictive models can forecast how different compounds will interact with the human body, significantly speeding up the drug development process. Researchers can use these models to simulate how different molecules might bind to specific proteins, making it easier to prioritize promising compounds for further testing.
AI-Powered Simulation and Experimentation for Clinical Trials
AI can accelerate scientific innovation by enabling simulation and virtual testing of hypotheses before physical experiments are conducted. This is especially useful in DeSci, where resources and access to labs are limited. Again, in drug discovery, AI can be used to model molecular interactions and predict the effectiveness of compounds before they are synthesized and tested in a lab. By using AI simulations, scientists can prioritize candidates for clinical trials and reduce the time and cost associated with drug development.
In other fields of traditional science, like materials science or energy research, AI can be used to simulate different conditions and predict the outcome of a research project. This way, researchers can explore more ideas and experiment with different approaches without the need for physical experiments.
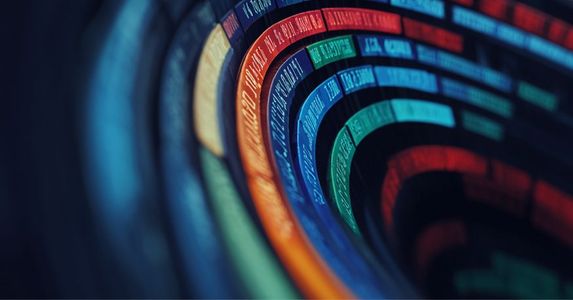
Peer Review, Research Integrity, and Intellectual Property
Peer review is an essential part of scientific research, ensuring published studies meet the highest standards of scientific research data and integrity. However, traditional peer review has its limitations, biases, delays, and lack of transparency. AI can enhance peer review by automating parts of the process and improving the detection of research and health data integrity issues.
AI can flag data inconsistencies, plagiarism, or methodology issues in academic or scientific publications that may go unnoticed during manual review. AI algorithms can analyze citation patterns in research papers to see if the work has been properly referenced and if the conclusions match the evidence.
In decentralized science, AI can further improve transparency by using scientific records and creating an immutable record of the review process on blockchain technology platforms. This ensures peer review is not only faster but also transparent and auditable, building trust in the scientific process.
Natural Language Processing (NLP) for Literature Mining
In decentralized science, researchers from all over the world contribute to a vast body of literature in the scientific field. However, with millions of scientific papers published every year, it can be challenging to keep up with the latest research findings and identify relevant studies for a particular project. AI-driven Natural Language Processing (NLP) is an effective tool for mining scientific literature and identifying key research findings first.
NLP algorithms can automatically extract key insights, relationships, and findings from various academic publications and research papers. These tools can scan thousands of research papers across multiple domains, identify the most relevant studies, and even summarize key insights. For decentralized science initiatives, NLP can help synthesize research from diverse disciplines and provide an overview of existing scientific knowledge, making it easier for researchers to collaborate and build on each other’s work.
Furthermore, AI can be employed to track trends in the scientific community and research, identifying emerging fields or promising areas for future investigation. This can help guide the direction of research and ensure that DeSci projects remain at the cutting edge of innovation.
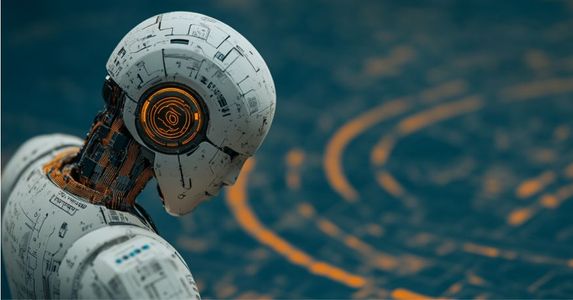
Ethical DeSci Will Rely on Ethical AI
As decentralized science (DeSci) continues to evolve, several ethical considerations must be addressed to ensure its success. One of the primary concerns is data privacy. With vast amounts of research data being shared across decentralized networks, it is crucial to protect sensitive information and ensure that researchers’ intellectual property rights are respected.
Another ethical challenge is the potential for biased or flawed research. DeSci platforms must incentivize researchers to produce high-quality, unbiased research. This can be achieved through transparent evaluation processes and fair compensation for contributions. To avoid biases and flawed research, DeSci will need to rely on ethical AI, and the best way to ensure transparency, privacy, and efficacy will be through decentralized AI models.
Decentralized autonomous organizations (DAOs) can also promote transparency and accountability in DeSci. DAOs can help manage research priorities and funding, ensuring that resources are allocated fairly and efficiently. Researchers and institutions must be aware of the potential risks and benefits of DeSci funding research initiatives and take proactive steps to mitigate any negative consequences. Decentralized AI models can also play a role in governance and funding protocols, helping to place ethical frameworks around DeSci operations.
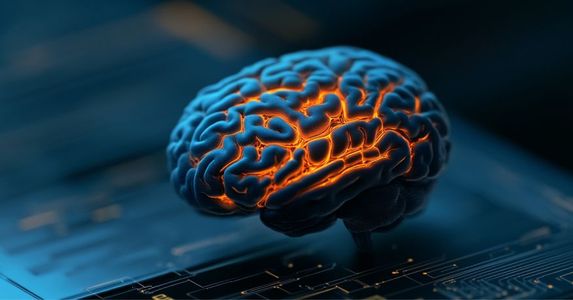
The Future of Decentralized Science: DeSci Powered by AI
The intersection of AI and DeSci has huge potential to change the way research is done, shared, and applied. From data analysis and interdisciplinary collaboration to research integrity and funding mechanisms, AI can facilitate scientific practices and supercharge DeSci’s potential.
As these technologies evolve, the collaboration between AI and DeSci can create a more inclusive, efficient, and transparent scientific ecosystem, accelerating science innovation and human knowledge.