We wouldn’t be able to envision the far-reaching, world-changing potential of DeSci without people like Stanley Bishop. Known as Science Stanley to many, Bishop has been an architect in the worlds of AI and machine learning for years, especially when it comes to creatively applying data to advance medical research.
Bishop contributes his AI expertise to a project called “Research to the People,” a joint effort between Stanford Genetics and Stanford Medicine that focuses on precision oncology and treating rare genetic illnesses. He’s also a research mentor for DeepChem, an AI-based chemistry project that utilizes open-source drug discovery tools and applies principles of multi-omics – a research approach that combines data from multiple scientific fields to give a more holistic view of scientific systems.
However, these are only two of the projects Bishop has contributed to. From facilitating AI collaboration with talented pianists to leading scientists over ocean horizons, Bishop proves that data science can be highly specialized but with broader and interwoven applications. DeSci Hub recently had the opportunity to speak with the “friendly neighborhood AI scientist” from his incubation studio in Venice Beach.
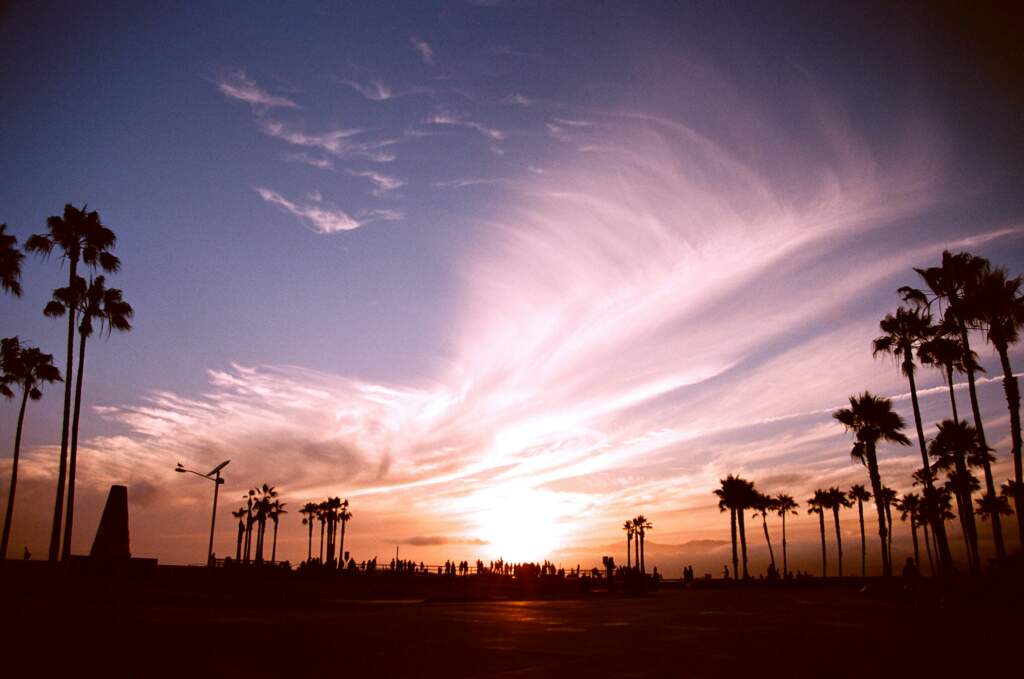
So, Venice? The very edge of Western Civilization …
I have to say, it is an interesting thing. It feels like we are just hanging here off the edge of the world, looking at where the sun sets. I’ve always really loved these kinds of creative bohemian enclaves. I was in Harvard Square before here, and then I was in Santa Cruz, California, before that. And, yeah, now Venice.
It’s my community. I love it. I think my approach to working with projects is very inspired by the ad hoc nature of community-driven projects. The incubator I’ve run here for about a decade is very much about growing projects out of the community.
You’ve been involved in a lot of projects, which we’ll get to, but your background is as a mathematician, correct?
Yeah, originally, right.
Does it all start with math?
For me, quite literally. If I’m working on a piece of AI code or a piece of systems architecture, or, quite frankly, even if I’m helping build a team, I view it as math. There’s a math subject called category theory, and in some sense, anything you study with math can be described in category theory.
I think, spiritually, the field of category theory does have this kinship to the field of decentralized science, because if you work in categories, if you take the categorical perspective, every field turns into just part of a bigger field. Category theory is like the ultimate decentralizer, and it definitely underlies both my career — my training as a category theorist in tech – and then specifically, I think it informs how I came to decide on a career path.
I was originally a principal investigator for Google, working in language localization, and it was funny because it was right before TensorFlow came out. A tensor is a pretty high-level math object. It’s a type of generalized number that really contains a lot of complex structure.
GPUs are all about processing tensors. That’s how most of machine learning works. I had that initial experience of, “You’re telling me all this stuff I was learning, which I thought was just for doing puzzles, is a multimillion-dollar subject?” It was an interesting experience for me, just as an individual. My journey from a mathematician to a technologist made me realize there’s so much cross-pollination.
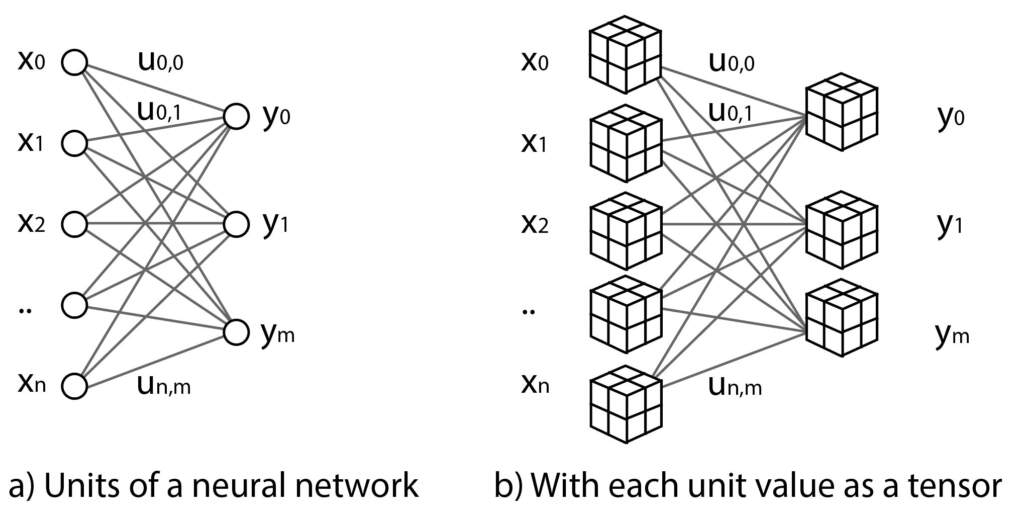
And this cross-pollination of tech, math, and data science can also be applied to other scientific fields, correct?
Yeah, especially in chemistry, but also biology. Most of the interesting work is very theoretically and mathematically driven, but I’ll admit something … I used to be very averse to biology and chemistry. I never really learned the subjects. I just memorized what I needed for tests.
It really was the arrogance of youth to not realize there was so much interesting stuff in those fields. I’ve been a research architect for a project called Deep Chem for six or seven years now. When I started contributing to that project, I knew so little about chemistry. The meetings were always a little nerve-wracking. I would just follow along, wait until they got to a part about math that was hard, and then I would help there and then.
But I also had this incredible experience of getting to learn and teach in an open-source community. It was how I was able to find my path. Math and data science seem at the core of many different fields of modern research, so I can imagine it’s cool to work in very interesting – but very different – fields of study.
Beyond any particular example, I think human culture and intelligence is most expressed when people are in really diverse, active settings. In my heart, I think of myself as a mathematician, even if I do work as a data scientist. But it’s a silly distinction.
Data science is so fascinating because I’m a data scientist who works with scientists, so I work with the data that scientists are using, and every scientific field has data. I can contribute to a project that’s deploying a Moon rover, which I’m doing right now. I can contribute to biology by helping to search for cures for rare illnesses. I can contribute to marine science and environmental protection.
We collect data on patients. We collect data on the oceans. The data itself and what we do with the data, that’s the field of data science. I actually tend to think of the work performed outside of the work we actually do as data scientists, but data scientists play a critical role. In many projects, we try to understand from the scientists what we need to know.
It always varies, but I do feel that beyond the technical utility, data scientists play a big role in helping integrate common knowledge because our goal is to understand the data from each direction. Since we might not always have the deepest picture from every direction, if we’re doing a good job, we’ll often find the best consensus picture.
How could data operate within a DeSci environment?
I think data plays a similar role in the broader ecosystem of DeSci, particularly because the blockchain is at its heart. It’s a consensus, democratically administered, and trustable database, right? Blockchain could naturally play such an important role in science.
We’re going through this crazy change where, all of the sudden, data is a much bigger deal. Having your data organized properly is like your ticket for participating in all this generative AI hoopla. It’s a really interesting time, and I’m very optimistic. In addition to being, in addition to doing important work, the broader data science community is contributing to a lot of fluidity and a lot of learning.
I often feel like the biggest role I play in an organization is helping the scientists learn from the technologists and the technologists learn from the scientists and aligning everything with the other leadership.
We collect data on patients. We collect data on the oceans. The data itself and what we do with the data, that’s the field of data science.
You’re also a passionate voice when speaking in DeSci spaces.
I do have a tendency to get up on my soapbox and share some of the things that I’ve seen in the centralized world that have made me really lean in the other direction. And, you know, it’s funny because, on a professional level, they encourage you not to share some of those, those harder realities.
But, you know, at some point, I feel like if we don’t start talking about that stuff … if we kind of have this conspiracy of silence in these fields, it’s how we get a system that we don’t feel comfortable with over time. I always share it with a positive vibe, but yeah, I really feel like this, especially in biotech. A lot of our leadership is really misaligned with our values as a people.
Can you speak a little on the concept of multi-omics?
One of the places where I’m able to create value is, as a mathematician, taking two subjects, which might seem very different, but when you describe them in math, you realize the math is the same. And sometimes the insights you get from recognizing that commonality are a big advantage.
A lot of my work revolves around multi-omics. You’ve probably heard about genomes and the Human Genome Project. Well, when the Human Genome Project was first completed, we all thought the world was going to change instantly. We thought the genetic code had all the answers. For example, we thought every disease is in the genome somewhere, and so for every disease, we’re going to be able to turn it off in the genome and cure the disease.
But, it turns out that genomics is just the start of the story. There’s the transcriptomics of how the DNA splits and replicates. There’s also the proteomics of how the RNA folds into proteomic proteins and shapes. There’s also the metabolomics of the chemical molecules that are created by proteins or that interact with proteins. And, so each of these layers has its own pretty incredible life of its own, and then the interactions between them is really where the big story is.
Can AI help with finding these bigger stories?
Yeah, absolutely. It turns out that a lot of the most intractable diseases and some of the diseases that would probably be the most impactful to cure are interactomics. Maybe you have a protein that’s malformed, but then that protein also interacts with a bunch of other chemicals and proteins in your body. Getting a clear picture of what happens in a disease condition is very challenging, but it’s something we’re just starting to be able to do.
Does this relate to your work with LabDAO?
Yeah, I was building open-source tools for this kind of work. One of the big initiatives was a diffusion docking system, which is a system that can take a molecule and a protein and kind of figure out how they fit.
The way a diff doc [diffusion docking] system works is all geometry, which is really a lot of fun. The same math you used to do Einstein’s theory of general relativity for black holes, you use to study the geometry of these proteins. And I think that’s so beautiful. It’s like the same tool applies to the biggest things.
… it turns out that genomics is just the start of the story.
Can you tell me a little about the startup studio?
Here in Venice Beach, I run an incubator that works with early-stage projects. I think you would love what we do. We like to find projects that normally wouldn’t get an enormous amount of support. And sometimes that means they’re creative projects, so there may be a musician or a sculptor who wants to use 3D printing or some kind of generative AI. It’s actually kind of fun.
Right now, we’re working with this incredible pianist. She’s so talented and has become very interested in using AI as like a collaborator, so she’s co-composing and playing different works with different AIs. We’re about to start building an AI for her inspired by the world’s genetic sequences. We’re going to take those environmental DNA sets and create a system that converts them to music that she can interact with. I would say most scientists are artists or musicians, and they view that part of their lives as deeply interwoven with their lives as scientists.
Can you speak a little about your work with cancer research?
One of the big areas I contribute to in oncology research is what is called an immune-cold tumor, which is a tumor that successfully evades the immune system. The tumor has figured out a way to totally mask itself from the immune system, and immune-cold tumors are very hard to treat. They don’t really react to chemo well, but if you can disrupt their obfuscation, sometimes the body will just kill them.
So, understanding a particular interaction that a tumor has with the surrounding tissue, figuring out how it’s disrupting detection, and then disrupting that disruption — sometimes that’s the cure. It’s like you’re just trying to find the right molecule that messes up what the tumor is doing. And when you introduce that molecule, it can be game over for the tumor.
Doesn’t this type of research kind of make you a de facto doctor?
Ha, no. I feel so privileged to learn the things that I learn, and I’m very humbled. I don’t know nearly as much as all the domain experts I work with, and I found that to be important to be successful in my role. I very specifically have to be humble and differential. Something I say in my talks a lot is: “A doctor must have good bedside manner. A machine learning scientist who works with doctors must also have really good bedside manner.”
I’m also going to recommend something to you. Check out this video. It’s a cartoon that explains the type of precision oncology that I work on. And it’s so fun. I think you’ll really enjoy it.
When you have a patient come in with immune-cold tumors, you need to figure out how to disrupt it. And it’s much easier to do if you have similar patients where doctors have tried some things. You can try to connect the dots between what happened in other patients. So, this is a big-data sharing challenge – a big challenge where decentralized technology could be the answer.
I have worked or work with physicians at Berkeley, Stanford, and USC. And they have an inter-institution data-sharing agreement, which is supposed to help convince scientists at each of those places to work on something together. But, even though they made that compact, it’s not like you don’t have to jump through any hoops. It’s just a hundred hoops instead of a thousand hoops.
Are there issues or concerns with sharing patient data?
It’s a tricky one, and there are some projects trying to make data freely shared. There’s a project I really like called The Cancer Genome Atlas, and I work with that data a lot, but there are some issues with the totally open sharing of patient data.
One thing a lot of physicians are really concerned about is that there are insurance companies that have started denying coverage to people who have disease biomarkers. So, let’s say you get cancer and you open source your genome, and then later, you apply for health insurance, but you get turned down because the insurance company found your genome online.
Is there a way blockchain could help with privacy?
In general, I think people should have control of their data, but I think we have a system that’s gone so far in the other direction. Even when you have patients, doctors, and scientists who all want to put the data together, you can’t get it done. I think blockchain is the solution to this, and it’s what I’m trying to work on right now. There are some new blockchain technologies that allow it.
You could get ten people to put their data together, but in a secure environment, so no one can actually see data that isn’t theirs. Once it’s in that environment, you use it to train an AI model, and then you delete the underlying data, so you get a model that doctors can use to treat patients that doesn’t actually expose any patient data.
You would also need to convince hospitals to use a system like this, but what percentage of hospital administrators do you think know about blockchain? There really is an educational problem, but, yeah, I think the solution to these data-sharing problems is 100% blockchain. It’s just a matter of building the tools, convincing the right people, and making it happen, but I am very excited. I think in the next six months or so, we’ll be training our first one of these models, and then we’re hoping it’s going to be used with patients at Cedars-Sinai this year.
Even when you have patients, doctors, and scientists who all want to put the data together, you can’t get it done. I think blockchain is the solution to this …
So, working with these hospitals with new tech is possible …
About ten years ago, I did a lot of work on a machine learning system for sepsis. We assembled a lot of patient data, and we figured out which patients became septic. And then we trained a machine learning model that could predict which patients were at risk of sepsis. We gave that to hospital administrators, and they were able to intervene when it looked like a patient was going to become septic.
In the hospitals where systems like this have been deployed, they’re preventing mortality from sepsis by 20% to 30%, and sepsis is a very common cause of death, so this is a pretty remarkable change. The thing that’s really crazy is that all this technology and this data was there 30 years ago. It shows the incredible damage we’re doing to patients just by having this kind of legacy system.
Last question. Along with everything else you’ve worked on, you’re also involved in ocean conservation research, correct?
Yes, there’s a punchline. I am very inspired by the reflection between human biology and the biology of the ocean. Among other things, all of our biochemistry evolved in the oceans, so in a very real way, the stuff that happens in us also happens in the ocean. And right now, we’re very scared about what will happen if the biodiversity of the ocean collapses.
The ocean produces half the oxygen we breathe, and it is possible that the whole biology of the ocean could crash if we push it too far. And it turns out that, on a biological level, the crash of biodiversity in an ecosystem is the same thing as sepsis. Sepsis is when the ecosystem of your body collapses.
So, it turns out that you can actually apply the exact same tools that you use to prevent sepsis to prevent biodiversity collapse in the ocean. And then, final punchline, (and this is so cool), since I shared with you about my journey as a mathematician coming to technology and science, I just finished leading a fellowship with this group called The Erdős Institute, and their whole mission is to take professors and research mathematicians and help them learn how to contribute to scientific technology projects.
So, I led a fellowship of around 30 PhD mathematicians who were each working on a different ocean and building one of these models to help understand the health of that ocean, and it went really well. We had our big demo day yesterday, and all the fellows presented the work they did. It’s all very inspiring. ***
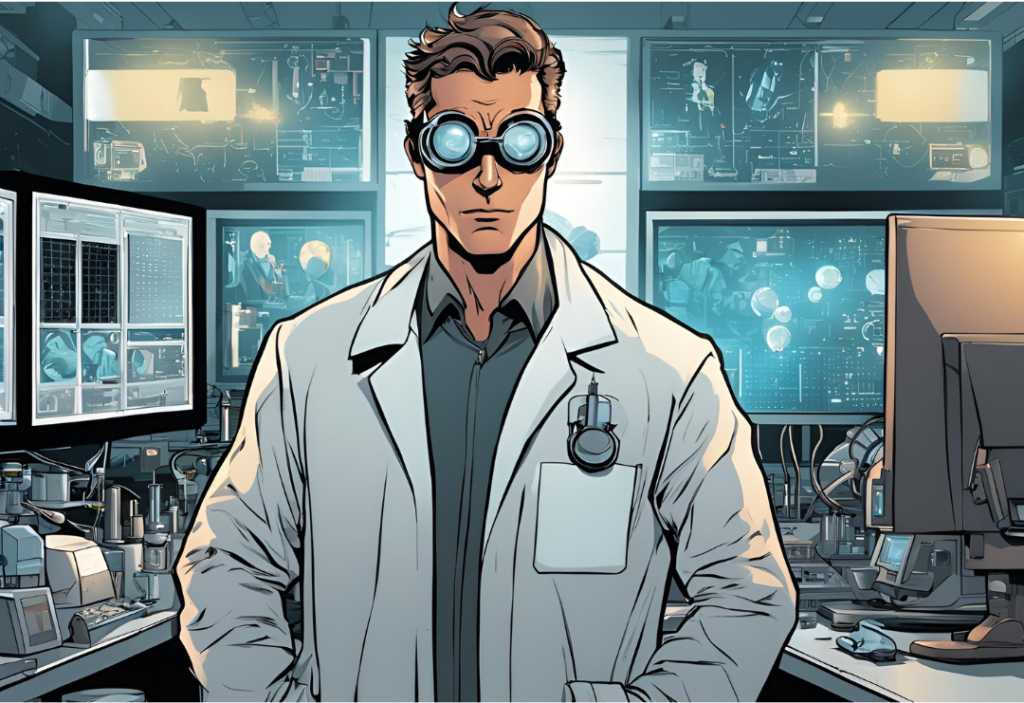
After speaking with Bishop, “inspiring” is a good word to end the interview with. As a data scientist, he was able to apply his work in sepsis to ocean biodiversity. As a free-thinking machine learning specialist, Bishop is facilitating collaborations between AI and artists in his Venice startup studio, and as a researcher in oncology, he’s assisting physicians to literally save lives.
Bishop is also a forward-thinking professional, utilizing Web3 to help create projects like LabDAO and DeepChem, which is an open-sourced library of tools for finding new medicines. According to Stanley, investors have laughed at the idea of open-sourced medicine discovery in the past, but as technologies continue to evolve and people like Bishop continue to push, this derision of the past is quickly giving way to the possibilities of the future. Inspiring, indeed.
It’s actually a great and useful piece of info. I am glad that you shared this helpful info with us. Please keep us up to date like this. Thanks for sharing.
Somebody necessarily lend a hand to make critically articles I’d state. That is the very first time I frequented your website page and so far? I amazed with the analysis you made to make this actual publish incredible. Fantastic process!